4 papers of IPEG researchers presented at the IEEE Power and Energy Conference at Illinois (PECI 2023)!
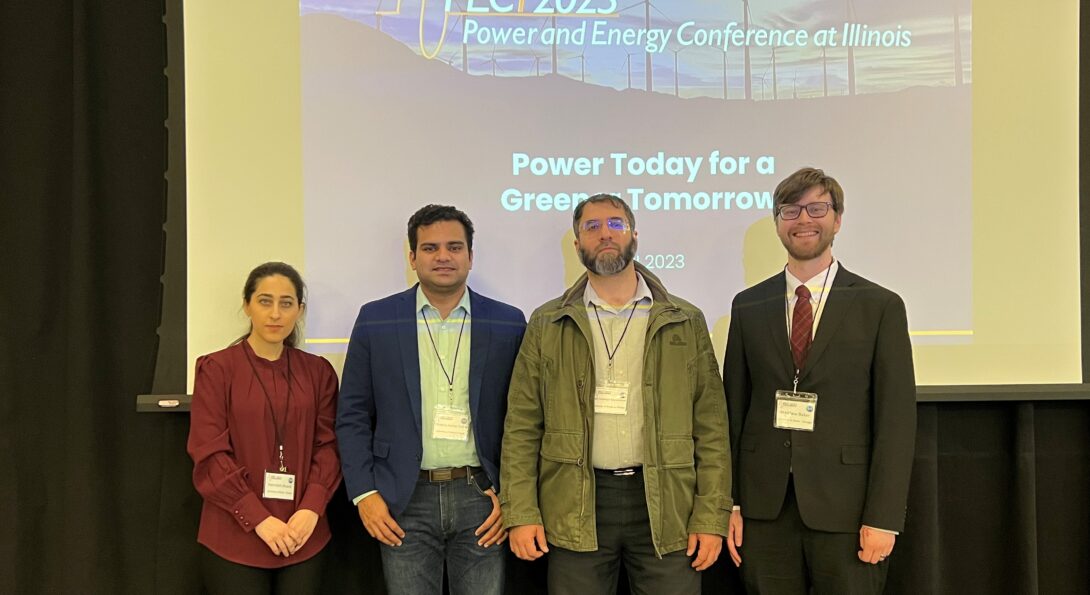
Introduction
IPEG researchers Hamideh Alvand, Silvanus D’silva, Matthew Baker and Asef Zadehgol-Mohammadi attended the IEEE Power and Energy Conference at Illinois (PECI 2023).
They presented 4 papers at PECI 2023 as the most recent research outcomes of IPEG lab. The papers focused on application of artificial intelligence and machine learning in inverter control approaches for applications in a power electronics dominated grid!
Paper 1: Data-Driven Based Corrective Actions and Detection of Intrusive Sensors Behavior in Power Electronic Dominated Grids
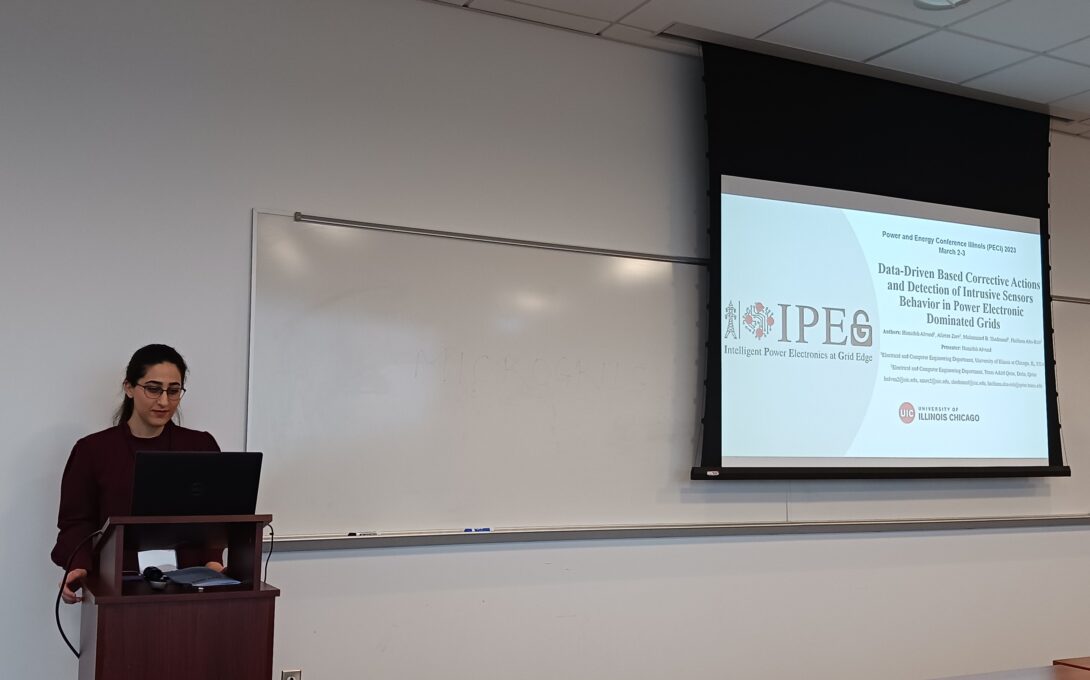
Abstract – This paper presents a Bayesian regularization based artificial neural network corrective actions for resilient operation of power electronic dominated grids (PEDG) with compromised sensors of grid-forming (GFM) or grid-following (GFL) inverters. GFM inverters play a significant role in governing the voltage and frequency stability of upcoming PEDG. Thus, spoofing the GFM inverter sensors’ readings for their feedback control systems can jeopardize the PEDG resilient operation. This paper presents a framework that corrects the spoofed sensors’ readings in the primary controller of the GFM inverter in PEDG. A neural network is trained via Bayesian regularization to realize the proposed corrective actions for sensors readings and consequently enhancing the resiliency of PEDG under sensor attacks. The trained neural network is integrated with GFM inverter’s primary control loop to provide real-time corrective action whenever intrusive sensor behavior is detected. Summary of the proposed framework is provided in this paper. The simulated case studies are provided in the paper to validate the theoretical expectations.
List of authors: Hamideh Alvand, Alireza Zare, Mohammad B. Shadmand, Haitham Abu-Rub
Presenter: Hamideh Alvand
Paper 2: Artificial Intelligence-based Frequency Trajectory Prediction to Enhance Power Electronics-Dominated Grid Resiliency
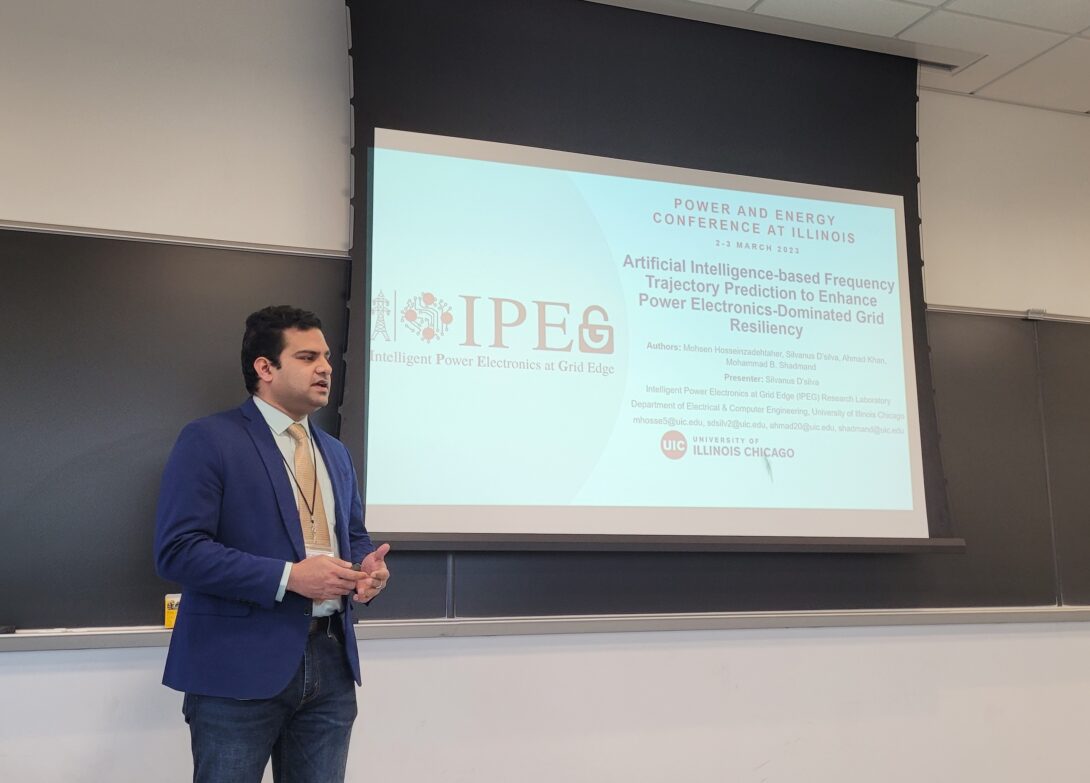
Abstract – This paper presents an Artificial Intelligence (AI)-based frequency trajectory prediction scheme to enhance power electronics-dominated grid’s (PEDG)s resiliency. The proposed approach incorporates two main modules: i) Data mining module, and ii) real-time AI-based frequency trajectory prediction module. The data mining module provides a comprehensive dataset for a wide range of system frequency and rate of change of frequency (ROCOF). This approach encompasses all practical ranges for total system inertia, vast range of load disturbances and system reactance-to-resistance (X/R) ratio. In this module, Bayesian regularization algorithm is used to compensate dataset size limitations, resolving noisy training dataset issues and cover any potential uncertainties. The AI-enabled neural network (ANN) module is incorporated in the grid following inverter’s (GFLI)s control loop to adjust their pre-defined power references in real-time during grid disturbances. The functionality of the proposed approach is validated via real-time testing of a 14-bus PEDG network on an OPAL-RT platform. Obtained results attest to the theoretical methodology validation and the robustness of the proposed approach.
List of authors: Mohsen Hosseinzadehtaher, Silvanus D’silva, Ahmad Khan, Mohammad B. Shadmand
Presenter: Silvanus D’silva
Paper 3: Deep Learning-Based Real-time Anomaly Detection for Power Electronics-Dominated Grid
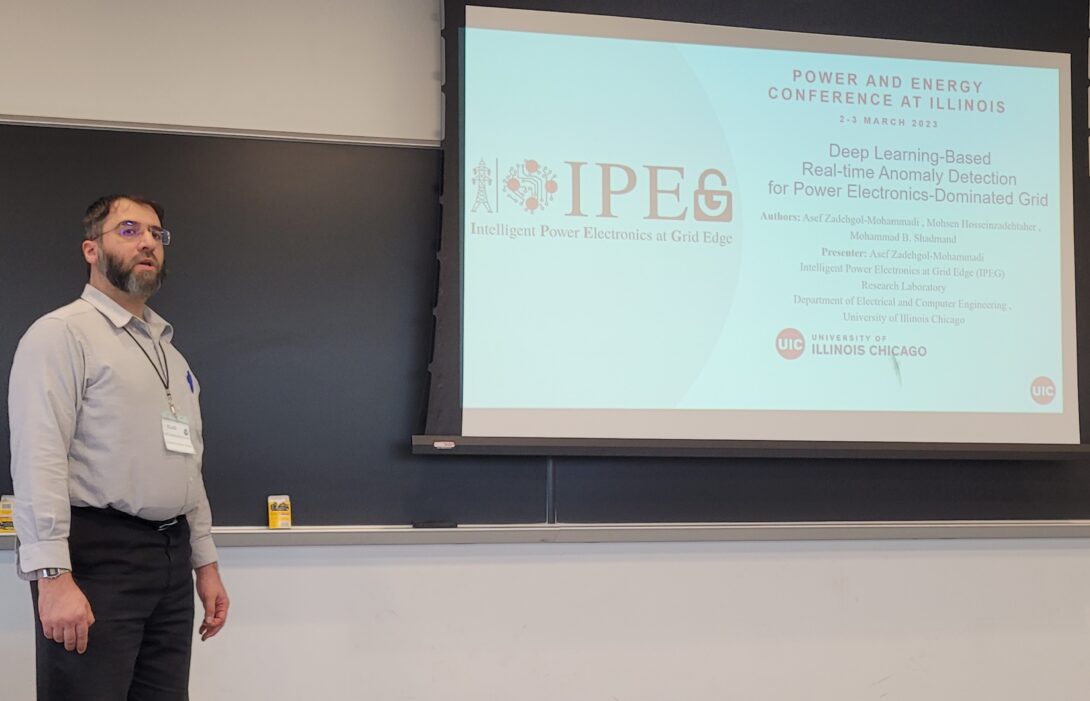
Abstract – This paper presents a type of recurrent neural network (RNN), namely, long short-term memory (LSTM) for real-time anomaly detection and classification at power electronics dominated grids (PEDG) inverters network. The proposed approach classifies signal sequences representative of voltage, frequency and rate of change of frequency (ROCOF) sensed at inverters point of common coupling (PCC), to normal or anomalous types. This paper addresses the challenges of training the network for real-time detection of anomalies in power grid due to large context of events. Numerous disturbances were included in the simulated PEDG for creating an effective database to avoid the neural network algorithm overfitting. The neural network classification accuracy and loss proved its capability of effectively detecting anomalous grid clusters with abnormal voltage and frequency behavior resulting from compromised inverters and their sensors. The preliminary case studies validate the performance and functionality of proposed real-time anomaly detection method for PEDG.
List of authors: Asef Zadehgol-Mohammadi, Mohsen Hosseinzadehtaher and Mohammad B. Shadmand.
Presenter: Asef Zadehgol-Mohammadi
Paper 4: An LSTM-based Anomaly Classification Framework for Power Electronics Dominated Grids
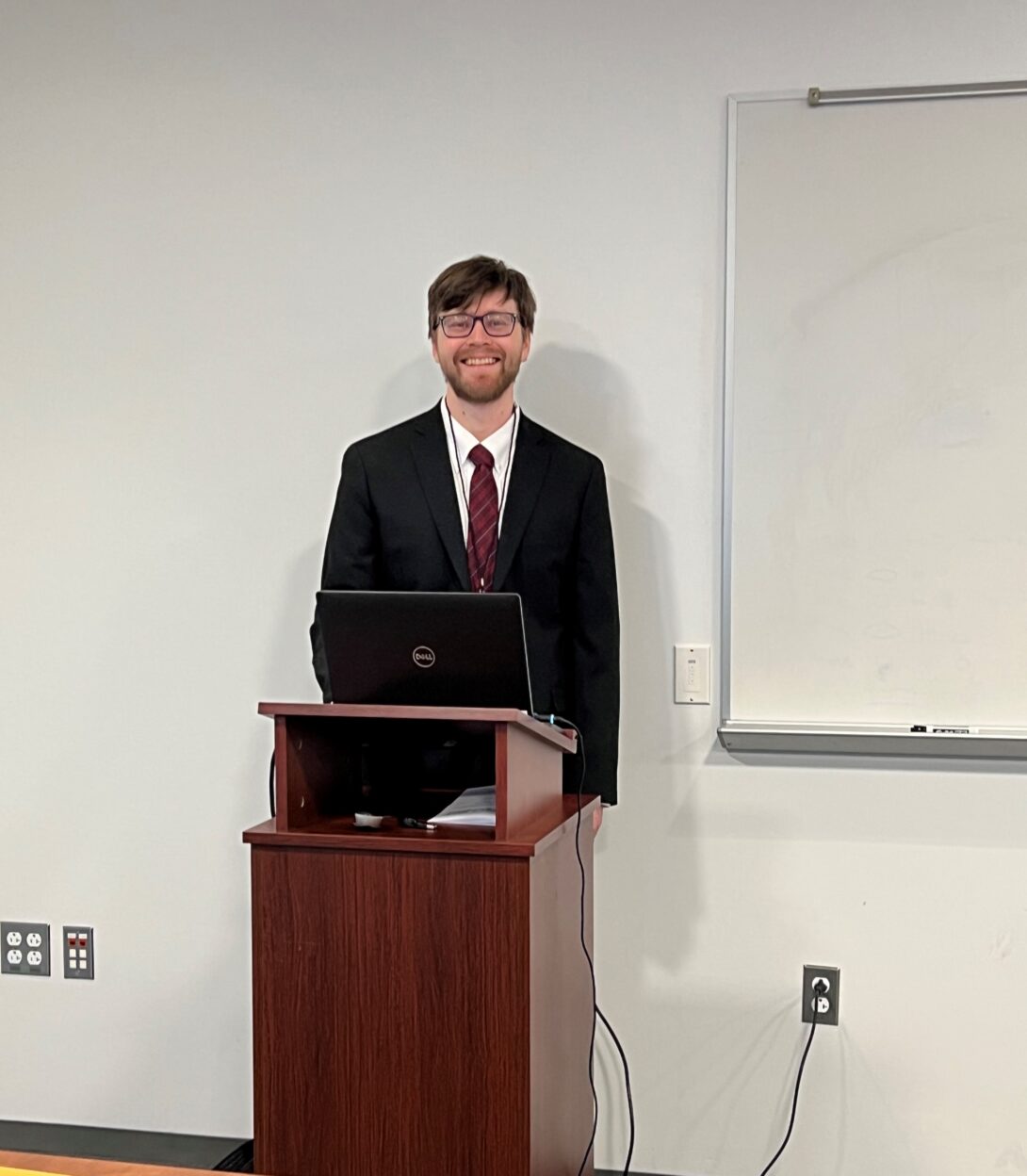
Abstract – The power electronics dominated grid (PEDG) is a paradigm shift which will see an increase of distributed energy resources (DERs) for power generation. As the PEDG becomes prevalent, grid anomalies must be detected and mitigated. Detection of these systems must be adaptive to changing grid topologies, and thus present an opportunity for data-driven solutions run by local and supervisory controllers in the PEDG. This work presents a line-to-line fault detection scheme built upon the long short-term memory (LSTM) neural network to determine quickly when line-to-line faults occur. The training process and data collection of the proposed line-to-line LSTM based fault detection can be extended to a multi-class classifier for detection of other anomalies in PEDG. The performance of the proposed LSTM based anomaly detection is validated via several case studies. The results demonstrate promising performance for real-time and effective anomaly detection in less than 20 ms to prevent catastrophic event in PEDG.
List of authors: Matthew Baker and Mohammad B. Shadmand.
Presenter: Matthew Baker