Amin Y. Fard successfully passed his Ph.D. dissertation preliminary examination!
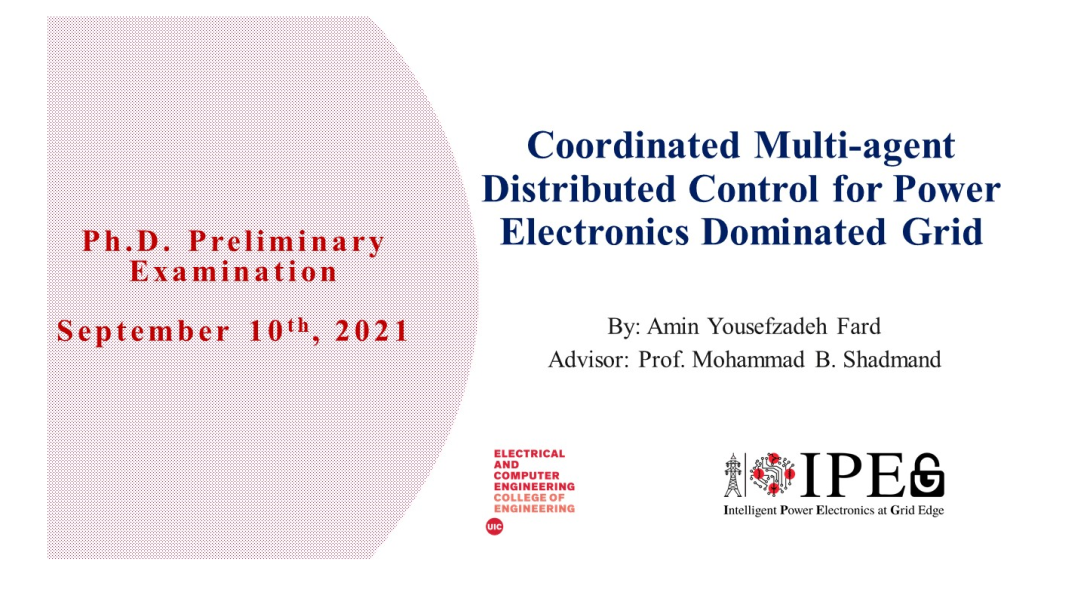
Introduction
Amin Y. Fard, Ph.D. candidate of the IPEG lab successfully passed his Ph.D. dissertation preliminary examination on 09.10.2021.
The title of his presentation was, "Coordinated Multi-agent Distributed Control for Power Electronics Dominated Grid."
The abstract of his presentation is as follows:
Abstract: Enhancing resiliency, improving stability, and ensuring the optimality of the power-electronics-dominated grid (PEDG) during various operating conditions are the crucial challenges that need to be addressed to facilitate the implementation of such a system. In this dissertation, a coordinated multi-agent distributed control framework is proposed. The proposed framework ensures the stability of the PEDG during frequency and voltage events for enhancing the resiliency of the entire system with minimized communications. First, a multi-timescale control framework is proposed, which has the capability of handling fast voltage-boundary violations in a short timeframe while optimizing the system operation in a slower timestep. The initial results prove that full-scale resiliency of the PEDG cannot be fulfilled if the controller handles voltage and frequency control, independently. To improve this aspect, in the second part of this dissertation, a cooperative multi-agent distributed control framework is proposed. Within the proposed framework, the PEDG is divided into clusters. Each cluster consists of one randomized super-agent and a couple of ordinary agents. This proposed framework reduces the traffic of the communication infrastructure significantly, since the ordinary agents only communicate with the randomized super-agent, while the super-agents of the clusters communicate with each other across the grid. Reduced communication yields to a more secure framework. In the case of any unexpected sudden changes i.e., load increase (a super-charger station connects to the grid), or loss of generation, the super-agent handles the demand in a short timeframe, while running a fast optimization to distribute the increased demand among the ordinary agents optimally, in a longer timeframe. The super-agents of the clusters, using the communication infrastructure correspond with each other to handle more severe events in a collaborative manner to ensure resilient operation. Recent cyberattacks on the power system of the United States raise the attention to look for some attack-resilient solutions for the power system. In the third portion of this dissertation, an attack-resilient control framework is proposed for the PEDG. Within this framework, the PEDG is divided into multiple clusters to ensure minimized communication during abnormal operating conditions. Each individual DER starts to estimate the impedance experienced at its point of common coupling via the proposed pseudo-random binary sequence impedance identification (PRBSII) method to begin behaving accordingly to maintain grid integration standards, and avoid islanding of DER, which would yield to cascading failures. Ultimately, using machine learning (ML) techniques, a grid clustering approach is proposed for the physical clustering of the DERs. Due to the inherent complexity of the PEDG alongside the abundance of data to be processed, ML-based techniques are the most-properly fitting option.