Mohsen Hosseinzadehtaher successfully passed his Ph.D. dissertation preliminary examination!
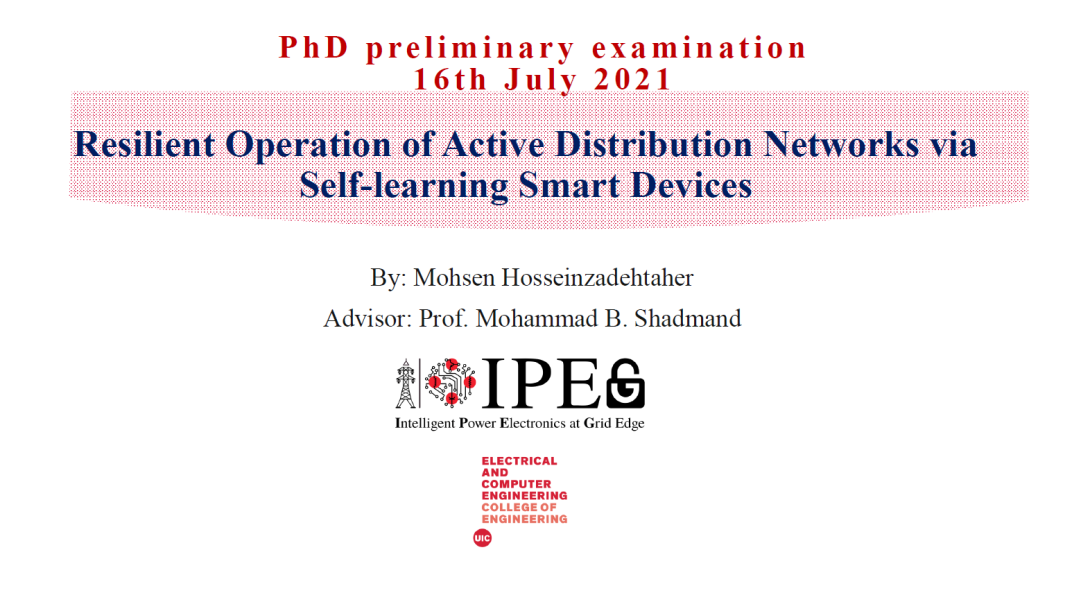
Mohsen Hosseinzadehtaher, Ph.D. candidate of the IPEG lab successfully passed his Ph.D. dissertation preliminary examination on 07.16.2021.
The title of his presentation was, "Resilient Operation of Active Distribution Networks via Self-learning Smart Devices."
The abstract of his presentation is as follows:
Abstract: This dissertation focuses on developing smart control techniques to enhance the resiliency of active distribution networks when potential disturbances occur. Self-learning smart devices play a crucial role for achieving the mentioned target. Fulfilling this goal, different control layers with different time scales have been designed and developed on a realistic 14- bus power electronic dominated grid (PEDG). The primary control layer improves the functionality of battery energy storage systems by employing a novel model predictive control scheme. This control can heal its operation to decrease the potential error in the controlling process. Moreover, the proposed control scheme improves the dynamic behavior of the charging and discharging process during load disturbances. The improved dynamics of the charging or discharging process can contribute to frequency restoration effectively. However, the frequency restoration process needs more advanced techniques. In the mentioned method, although frequency restoration is improved but it is not the optimum one. Therefore, it is necessary to develop an artificial inspired technique to realize a self-learning control scheme with ultra-fast frequency restoration in the network.
An artificial intelligence-inspired model predictive control has been developed in this work which uses a two-layer feed-forward neural network. This artificial neural network is trained to provide a real-time optimum active power during grid disturbances. For providing the training data, fundamentals equations that are related to synchronous generator swing calculations are studied. This equation are solved based on a wide range of load disturbances and system inertia constants and feasible solutions congregated as training data. Moreover, the required power for each scenario is used as the target data. A Bayesian algorithm was employed to improve the training performance of the neural network. Generally, in this approach, frequency, and rate of change of frequency (ROCOF) are considered in the training process. This idea improves the frequency restoration process in a better and faster way. The proposed method was evaluated on the 14 bus PEDG and the results attested to the promising functionality of the proposed technique. To assess the proposed technique’s functionality in a realistic condition, a hardware setup was developed. In this setup, two three-phase inverters have been used. Inverter controlling cores are based on the model predictive control approach. To mimic the behavior of the synchronous s generator in our experiment, one of the inverters was operated as the grid forming and the other one followed the grid forming inverter. Hardware test results have shown that, by triggering the ANN module in the proposed technique, frequency is restored fast and smooth. Taking into consideration that in a relatively big network such as our system, it is crucial to evaluate results in a real-time simulation and observe the real dynamic of the system parameters as well as their complex interaction in a real-time system. The 14-bus system model was built in an OPAL-RT simulator and the results proved the promising functionality of the ANN, particularly during the grid’s disturbances. The proposed control technique which takes advantage of the ANN’s features can be used as a shadow controller in the supervisory control layer too.
In the new energy paradigm, cyber-attacks are known as a dangerous phenomenon that can easily make unstable the entire network if not detected or mitigated promptly. Therefore, the proposed control technique was used for the case that the attacker tries to unstable the entire grid via stealthy attacks. Stealthy attacks are done in a way that state variables are diverged before observable global variables. Therefore, they can jeopardize system’s demand-supply balance if not detected and controlled in a short time. Some stealthy attack scenarios were analyzed in this dissertation and the designed shadow control supported the grid frequency to be restored even when a large generation loss occurs. Regarding the device level of cyber-attack aspects, false data injection (FDI) attacks are more common. They can manipulate the measurements in different grid access points. For having a more resilient control framework, EMI attacks were surveyed in this work too. For emulating the EMI attack, a model of white noise was applied on the inverter's current sensors and the measurement was manipulated. The results show that if the noise energy is relatively high, system stability can be endangered. The main effect of EMI attacks is reflected in the frequency and ROCOF and this is due to the manipulated inverters which are injecting commanded active power to the main grid. It worth mentioning that, in a normal and safe operation of a grid, several normal load disturbances occur which result in frequency excursion. However, the inherent pattern of these fluctuations differs from the EMI ones. In this work, a deep learning-based proactive intrusion detection mechanism for a power electronics-dominated grid was developed which can detect the normal fluctuations and EMI attacks during the disturbances. Long short-term memory (LSTM) neural networks which are type of recurrent neural network (RNN) are used to classify signal sequences. Each time the detection module attest that an EMI attack has occurred, the inverter current sensor data will not be used anymore, and a well-designed observer will be activated to support the grid operation till the supervisory layer testifies the attack has been removed from the entire system.
Based on the importance of the power grid stability, it is crucial to have another layer of control which monitors the grid dynamic and vital information in other time scales. For any reason, if any of the other control layers fail, this layer will take proactive action to maintain system stability. The groundwork of this control layer is based on the load forecasting technique. In this work, an anomaly detection technique in a distribution power system based on a condition monitoring vector and ultra-short demand forecasting is designed and developed for achieving the mentioned goal. Temperature data and historical loads are the input of this technique. According to the safe level of historical data and temperature data, this detection technique is more robust against attack scenarios. The supervisory layer can forecast the load in a short duration several times across the network and compare it with real-time data. The designed condition monitor vector acts based on a defined upper and lowers bound. These bounds are defined based on the acceptable and inherent tolerance in forecasting and measurement and will be able to make the final decision to isolate an area, flag an area or confirm the safety level of grid operation.