Prof. Shadmand and Dr. Farooq attended the 4th International Conference on Smart Grid and Renewable Energy (SGRE 2024), Doha, Qatar from Jan 8-10, 2024.
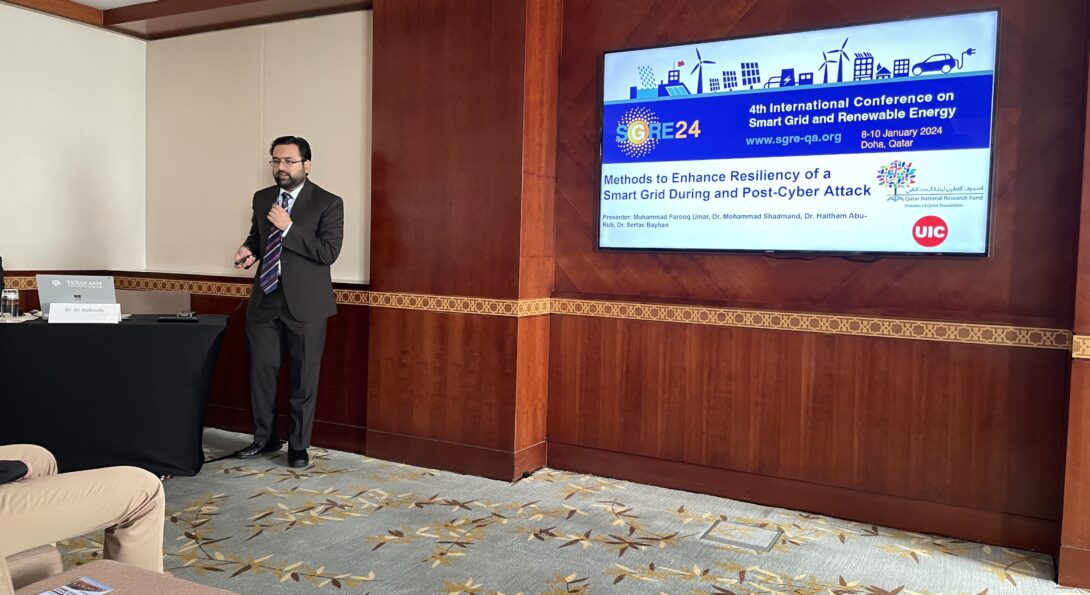
Prof. Shadmand and Dr. Farooq attended the 4th International Conference on Smart Grid and Renewable Energy (SGRE 2024), Doha, Qatar from Jan 8-10, 2024. During the conference, Dr. Farooq presented three papers discussing the most recent research outcomes of IPEG lab. These papers focused on applications of artificial intelligence in fault detection and localization, challenges in inertia emulation, and learned state-space model based zero-dynamics stealthy attacks in a power electronics dominated grid!
Paper 1: Prospects and Challenges of Inertia Emulation Methods for Low Inertia Power Systems
Abstract – Due to the massive incorporation of renewable energy resources into the upcoming power grid, the share of power generation from synchronous generators (SG) is being decreased and is being replaced by distribution generation (DG). This change has led to a significant decrease in the overall power system’s inertia and led to transforming the future power system into low inertia power systems (LIPS). Because of the huge rotational mass of SG, it intrinsically provides system inertia that is critical for the stable and resilient operation of the power system under different types of disturbances. To cope with the challenges of stable operation of LIPS, several inertia emulation schemes have been proposed in the previous literature. However, each scheme has its specific benefits, limitations, and applications. Thus, this paper provides a comprehensive insight into the inertia emulation schemes for DG and compares each scheme in terms of implementation challenges and experimental effectiveness in enhancing the overall system’s inertia. Moreover, this comparison is supported by experimental results and analysis to validate virtual inertia emulation schemes.
List of Authors: Muhammad Farooq Umar, Amirhosein Gohari Nazari, Mohammad B. Shadmand
Presenter: Muhammad Farooq Umar
Paper 2: Real-time AI-based Fault Detection and Localization in Power Electronics Dominated Grids
Abstract – This paper presents a real-time fault detection and classification network for power electronics-dominated grids (PEDG). The challenges in the detection and localization of faults in active distribution networks are addressed by the proposed approach. The proposed approach is based on a long short-term memory (LSTM) neural network to detect and localize faults based on measurements at the point of common coupling of distributed energy resources (DERs) within the network. The proposed scheme is implementable at the grid edge in active distribution networks for real-time detection, classification, and localization using DERs as a grid probing tool to enhance the situational awareness of futuristic PEDG. This work includes a detailed theoretical analysis and case study that evaluates the performance of the proposed LSTM-based fault detection and localization in active distribution networks. A comprehensive database is created for the training process and the network operates with optimized hyperparameters. The proposed method is examined for a modified IEEE 14-bus network dominated by DERs. The results demonstrate strong performance and fast (i.e., within one line cycle) fault detection and localization that enhances the situational awareness of the system.
List of Authors: Matthew Baker, Muhammad Farooq Umar, Mohammad B. Shadmand, Arslan Munir
Presenter: Muhammad Farooq Umar
Paper 3: On Zero-Dynamics Stealthy Attacks with Learned State Space Models
Abstract – Zero-dynamics stealthy attacks are a subset of false data injection attacks (FDIAs) that can be catastrophic as they are designed to be undetectable by traditional residual-based anomaly detectors. The attacker’s ability to successfully attack a system relies heavily on their capacity to learn an accurate state space model. Utilizing a grey box approach to system identification, we show that even when the attacker can learn a state space model close enough to have a high probability of a successful attack, making small improvements to the system’s anomaly detector causes the probability of a successful attack to drop drastically. Finally, we study the trade-offs between making the system less susceptible to zero-dynamics attacks and maintaining its controllability, by increasing the sampling time of the system, thus providing the attacker with fewer samples to learn a state space model.
List of Authors:Stephanie Harshbarger, Bala Natarajan, Eugene Y. Vasserman, Muhammad Farooq Umar, Mohammad B. Shadmand, George T. Amariucai
Presenter: Muhammad Farooq Umar